Understanding the Environmental Impact of AI Research
What is AI and its Role in Environmental Issues?
Defining Artificial Intelligence
Artificial Intelligence (AI) refers to a branch of computer science focused on creating systems capable of performing tasks that typically require human intelligence. This includes problem-solving, understanding language, recognizing patterns, and making decisions. AI technologies are increasingly integrated into various sectors, including healthcare, transportation, and energy management, demonstrating their transformative potential.
The Dual Nature of AI: Problem Solver vs. Environmental Burden
While AI holds promise as a tool for tackling environmental challenges, such as optimizing energy use and monitoring wildlife, its development raises significant concerns about its environmental footprint. The technology's infrastructure, primarily data centers, consumes vast amounts of energy and contributes to carbon emissions, thus presenting a paradox where AI can be both a solution and a source of pollution.
The Carbon Footprint of AI Research
Energy Consumption in AI Development
Training Large Models: The Energy Drain
Training large AI models is an energy-intensive process. For instance, according to a study from the University of Massachusetts, training a single AI model can produce emissions equivalent to five cars over their lifetimes. As datasets grow larger and algorithms become more complex, the energy needed for training continues to escalate.
Inference Costs and Ongoing Usage
Once AI models are deployed, their energy consumption doesn't cease. Inference—the process of making predictions based on trained models—can require even more energy than training. AI systems like ChatGPT reportedly consume significant electricity, leading to an increased carbon footprint during everyday usage.
Comparison of AI's Carbon Emissions to Other Industries
The carbon emissions from AI development are staggering, with data centers responsible for approximately 2.5 to 3.7% of global greenhouse gas emissions—surpassing those of the aviation industry. This highlights the urgent need for sustainable practices within the AI sector to mitigate its environmental impact.
Electronic Waste and Sustainable Practices
E-Waste Generated by AI Technologies
The rapid advancement of AI technologies also results in considerable electronic waste (e-waste). Many components of AI systems, such as GPUs and servers, contain hazardous materials like lead and mercury. Improper disposal can lead to serious environmental contamination and health risks.
Hazardous Materials and Disposal Challenges
E-waste management poses challenges due to the toxic substances it contains. The World Economic Forum projects that by 2050, global e-waste could exceed 120 million metric tons. This highlights the necessity for effective recycling and disposal strategies to minimize environmental harm.
Strategies for Reducing E-Waste
Responsible Recycling and Disposal Methods
To tackle e-waste, it's essential to implement responsible recycling and disposal methods. Encouraging manufacturers to design products with recyclability in mind can significantly mitigate the e-waste crisis.
Innovations in Sustainable Hardware Design
Innovations in hardware design can also play a significant role in reducing e-waste. Companies like NVIDIA are investing in sustainable GPU development and promoting recycling initiatives, paving the way for greener technology.
The Role of Sustainable AI Practices
What is Sustainable AI?
Definition and Importance
Sustainable AI encompasses practices that prioritize energy efficiency and reduced environmental impact in AI systems. This approach aims to ensure that AI technologies contribute positively to society while minimizing their ecological footprint.
How Sustainable AI Differs from Traditional AI
Unlike traditional AI, which often emphasizes performance over sustainability, sustainable AI incorporates eco-friendly practices from the design stage through deployment. This includes optimizing algorithms for energy efficiency and utilizing renewable energy sources in data centers.
Examples of Sustainable AI Practices
Energy-efficient Algorithms and Models
Research into energy-efficient algorithms aims to reduce the computational power required by AI systems. Techniques such as pruning models to eliminate unnecessary parameters can lead to substantial energy savings without sacrificing performance.
Green Data Centers and Their Impact
Green data centers utilize renewable energy sources and implement energy-efficient cooling systems. Companies like Google are leading the charge, reportedly running their data centers on 100% renewable energy, thus significantly reducing their carbon footprint.
The Impact of Machine Learning on the Environment
How Machine Learning Contributes to Environmental Pollution
The Water Footprint of Data Centers
Data centers not only consume large amounts of energy but also require significant water for cooling purposes. This dual demand exacerbates environmental pressures, particularly in regions facing water scarcity.
Mining for Rare Earth Elements
AI technologies often rely on rare earth elements, which are mined through environmentally damaging processes. The extraction methods can lead to habitat destruction and pollution, raising ethical concerns about the sourcing of materials used in AI hardware.
Balancing AI for Environmental Improvements
AI in Climate Monitoring and Mitigation
Despite its drawbacks, AI can be instrumental in addressing climate change. For example, AI applications in climate modeling can enhance predictions, enabling better preparedness for environmental impacts.
Case Studies of AI Helping the Environment
Numerous case studies demonstrate AI's potential in environmental conservation efforts. From monitoring deforestation to optimizing resource use in agriculture, AI can help mitigate some of the adverse effects of climate change.
Future Directions for Green AI
Emerging Technologies and Their Potential
AI in Renewable Energy Management
AI technologies can optimize the management of renewable energy sources, improving efficiency and reducing waste. By accurately predicting energy needs, AI can facilitate better resource allocation and lower carbon emissions.
Innovations in Smart Grids and Resource Efficiency
Smart grids powered by AI can enhance energy distribution and reduce consumption through real-time data analysis. This technology is pivotal for transitioning to more sustainable energy systems.
Regulatory Frameworks and Their Importance
Current Policies and Recommendations for Sustainable AI
Governments and organizations are beginning to recognize the need for regulations that promote sustainable AI practices. Establishing standardized measures for assessing the environmental impact of AI can foster greater accountability.
The Need for Transparency and Accountability in AI Development
Transparency in AI systems is crucial for understanding their environmental impact. Companies must disclose their energy consumption and emissions data to enable informed decision-making and drive sustainable practices.
Conclusion
Summary of the Environmental Impact of AI Research
AI research presents a complex relationship with the environment. While it offers solutions for pressing ecological challenges, its development and deployment contribute significantly to carbon emissions, e-waste, and resource depletion.
The Path Forward: Integrating AI and Sustainability Practices
Moving forward, integrating sustainable practices into AI research is imperative. By prioritizing energy efficiency and responsible resource management, we can unlock AI's potential while safeguarding our planet for future generations.
Key Takeaways
- AI has both positive and negative environmental impacts, serving as a tool for conservation yet contributing to pollution.
- Training and using AI models consume significant energy, with emissions comparable to multiple cars over their lifetimes.
- E-waste from AI technologies poses serious environmental challenges, necessitating improved recycling and sustainable hardware design.
- Sustainable AI practices focus on energy efficiency, promoting greener algorithms and renewable energy in data centers.
- Regulatory frameworks are essential for ensuring transparency and accountability in AI's environmental impact.
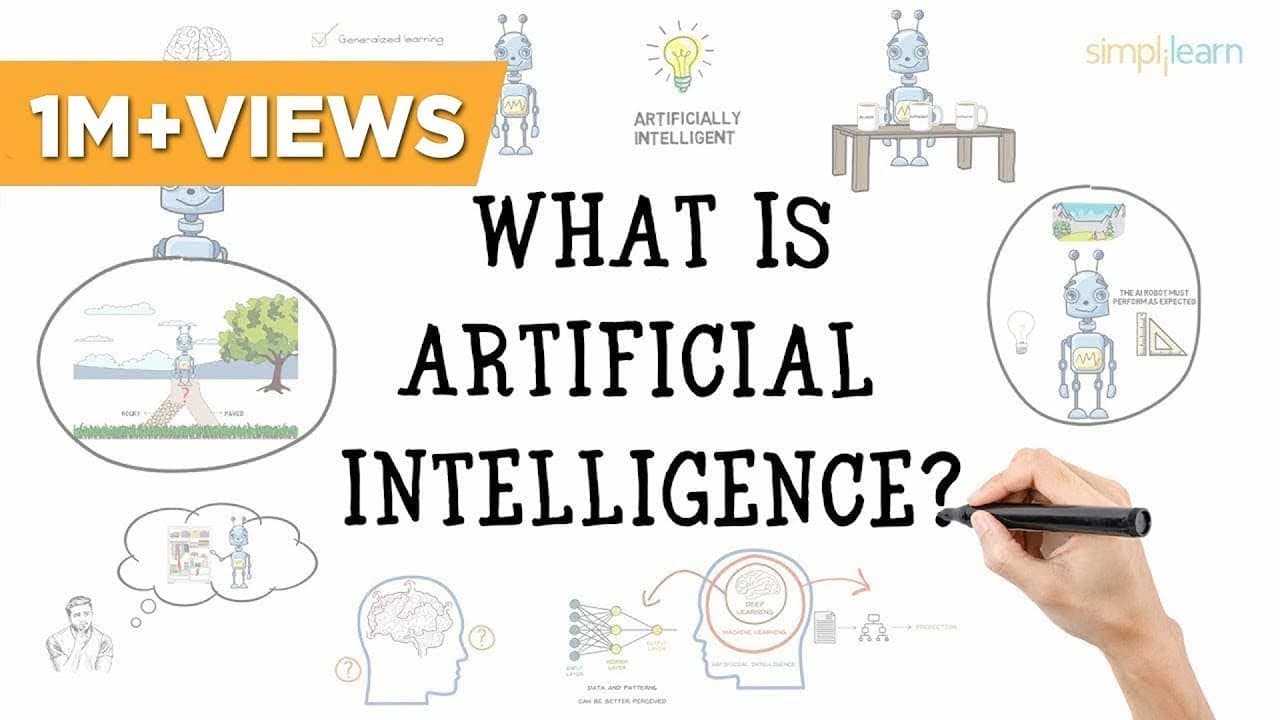