Understanding ChatGPT's Carbon Emissions
Overview of ChatGPT's Energy Consumption
ChatGPT is a powerful tool, but it needs a lot of energy. This energy use contributes to its carbon footprint.
It's estimated that ChatGPT consumes over half a million kilowatts of electricity each day. This is comparable to servicing about two hundred million requests daily.
Daily Carbon Footprint of ChatGPT
ChatGPT generates an estimated 8.4 tons of CO2 annually. This is double the average person's yearly output.
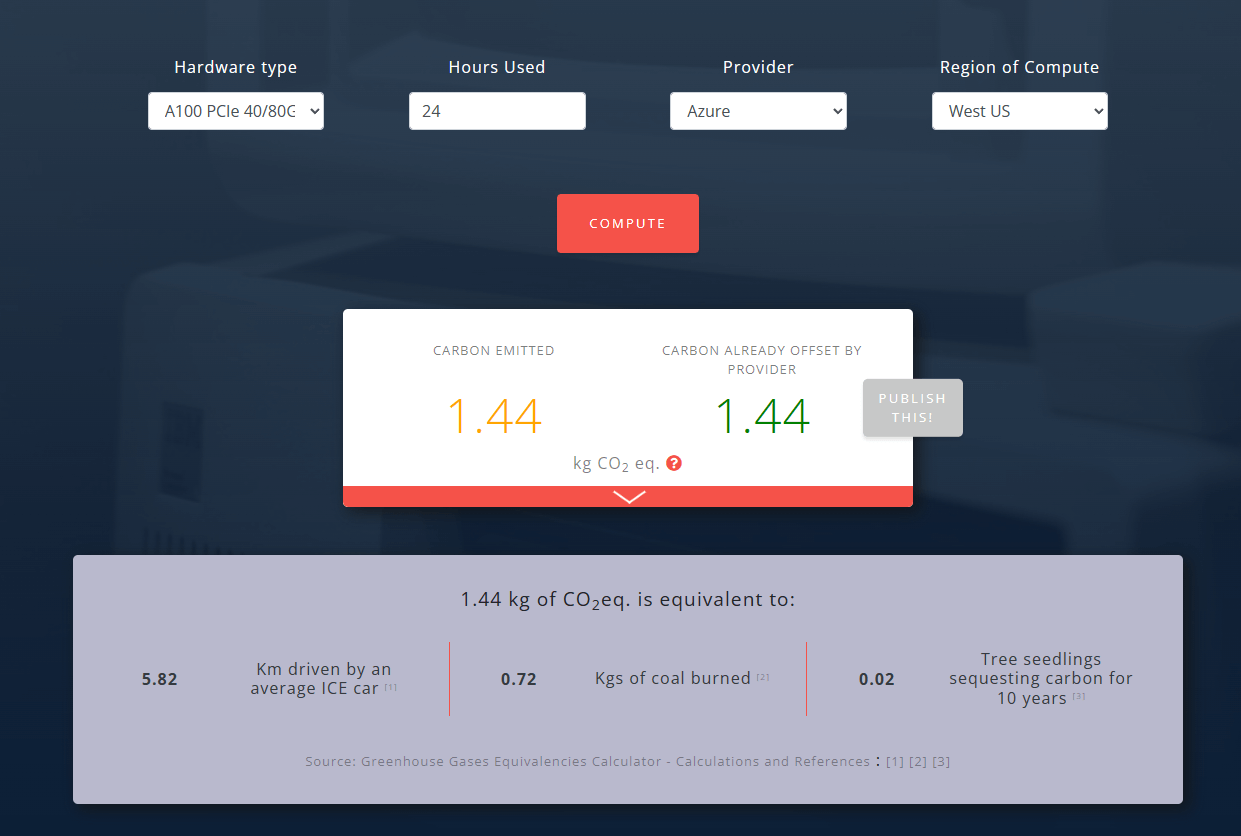
These initial calculations assumed ChatGPT ran on 16 GPUs. The reality is a staggering 30,000 units, if not more, meaning the true carbon cost could be much higher.
Annual Emissions Compared to Average Individual
An average person emits about 4 tons of CO2 per year. ChatGPT's emissions are more than double that amount.
This highlights the significant environmental impact of AI models.
CO2 Emissions per Query
Each query to ChatGPT produces approximately 4.32 grams of CO2. It might not sound like much.
However, with millions of queries daily, the numbers quickly add up. For example, 15 queries are equivalent to watching one hour of videos, and 16 queries are like boiling one kettle.
The Environmental Impact of AI Models
Energy Demands of Data Centers
Data centers that power AI models like ChatGPT consume vast amounts of energy. These facilities house power-hungry servers required by AI models.
They generate a substantial carbon footprint. Cloud computing, used by AI developers like OpenAI, relies on chips inside these data centers to train algorithms and analyze data.
Water Usage in AI Operations
AI models, including ChatGPT, have a significant water footprint. During GPT-3's training, Microsoft used around 700,000 liters of freshwater.
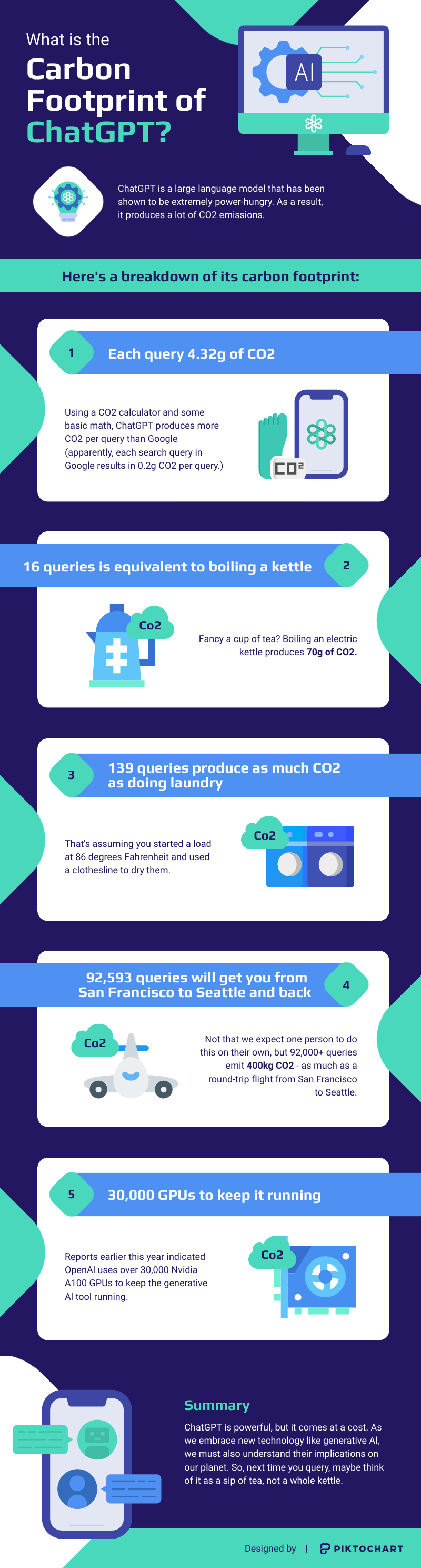
This is similar to the amount of water needed to produce 370 BMW cars or 320 Tesla vehicles.
Comparison to Traditional Manufacturing
Training ChatGPT might use as much water as manufacturing 370 BMWs or 320 Teslas. The water usage is primarily due to the need to cool down machinery during the energy-intensive training process.
A simple conversation of 20-50 questions with ChatGPT consumes water equivalent to a 500ml bottle. This consumption adds up with billions of users.
E-waste and Material Consumption
Data centers produce electronic waste. The electronics rely on a staggering amount of raw materials.
For example, making a 2 kg computer requires 800 kg of raw materials. The microchips that power AI need rare earth elements, often mined in environmentally destructive ways.
Calculating ChatGPT's Carbon Footprint
Methodology Behind Emission Estimates
The exact number of GPUs used by ChatGPT is obscure. Reports indicate an estimation of 30,000 GPUs per day.
Each GPU, when run continuously over 24 hours, emits 1.44kg of CO2 per day. These figures help us understand the scale of emissions.
GPU Usage and Their Carbon Output
OpenAI uses enterprise-grade A100 GPUs. These are 5x more energy efficient than CPU systems for generative AI applications.
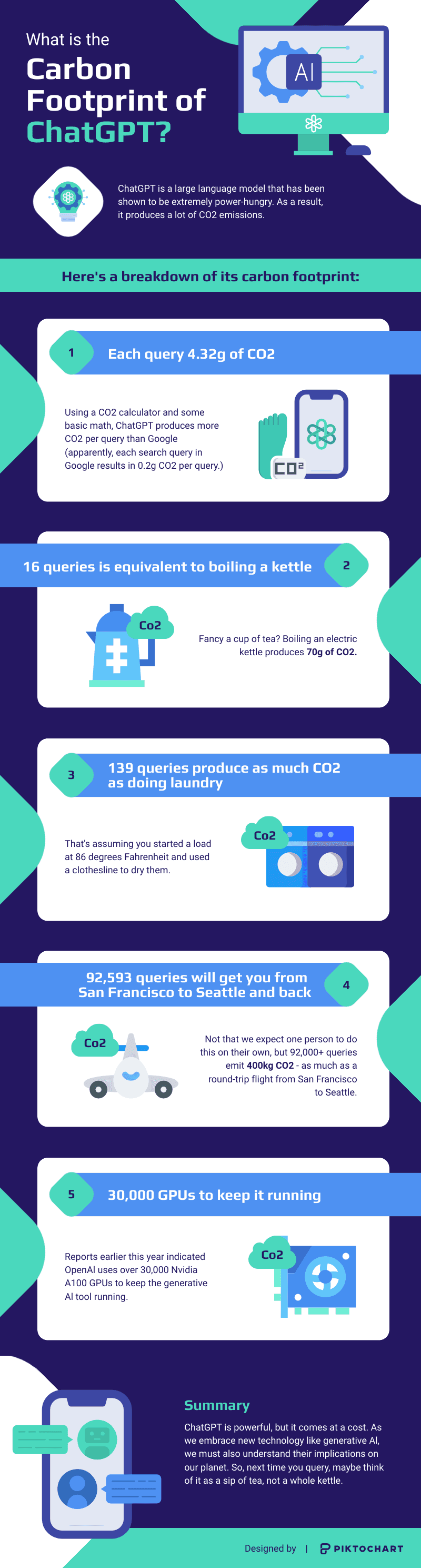
However, with 30,000 GPUs in use, that means 43,200kg CO2 is being emitted daily.
Inference vs. Training Energy Requirements
Training GPT-3 consumed 1287 MWh of electricity. This resulted in carbon emissions of 502 metric tons.
Inference, where the AI responds to queries, may consume even more energy than training. Google estimated that 60% of the energy used in AI goes towards inference.
Comparative Analysis with Other AI Systems
Google's search engine primarily retrieves and ranks existing web pages. ChatGPT generates new responses based on training from vast datasets.
This process, known as inference, demands much higher computational power. Consequently, it requires more energy.
Strategies for Reducing Carbon Emissions in AI
Energy Efficiency Improvements
Companies are focusing on optimizing hardware and improving algorithms. Advances in energy-efficient chips and data center cooling techniques can help.
Software optimization may also reduce energy demand over time.
Advances in Hardware and Software Optimization
OpenAI is exploring the development of their own energy-efficient chips. They are also using Microsoft's Azure cloud provider, which is carbon neutral.
These efforts show a commitment to lowering their carbon footprint.
Transitioning to Renewable Energy Sources
Microsoft is committed to running on 100 percent renewable energy by 2025. Google's data centers already get 100 percent of their energy from renewable sources.

Moving large jobs to data centers with clean energy grids makes a big difference. This transition is crucial for reducing the environmental impact of AI.
Promoting Transparency in AI Development
Scholars have developed frameworks to assist researchers in reporting their energy and carbon usage. There are online tools that encourage teams to conduct trials in eco-friendly areas.
These tools provide updates on energy and carbon measurements. They actively assess the trade-offs between energy usage and performance before deploying energy-intensive models.
Future Outlook on AI and Sustainability
Potential Role of AI in Environmental Conservation
AI can help monitor the environment and predict future outcomes. For example, it can be used to detect when oil and gas installations vent methane, a potent greenhouse gas.
AI can also enhance efficiencies in various sectors.
Innovations to Minimize AI's Environmental Impact
Researchers are trying to create language models using smaller data sets. The BabyLM Challenge aims to get a language model to learn from scratch like a human.
This approach reduces the time and resources needed for training. Smaller models consume less energy.
The Importance of Policy and Regulation in AI Development
Over 190 countries have adopted non-binding recommendations on the ethical use of AI. These recommendations cover the environment.
The European Union and the United States have introduced legislation to temper the environmental impact of AI. However, more policies are needed.
Key Takeaways:
- ChatGPT's daily carbon footprint is substantial, with each query producing about 4.32 grams of CO2.
- Data centers powering AI consume vast amounts of energy and water, comparable to traditional manufacturing.
- Transitioning to renewable energy sources is crucial for reducing AI's environmental impact.
- Improving hardware and software efficiency can significantly lower energy consumption.
- Promoting transparency and developing supportive policies are essential steps for sustainable AI development.